Color Theory in Research Visualization: Best Practices
November 17, 2024 | by Jean Twizeyimana
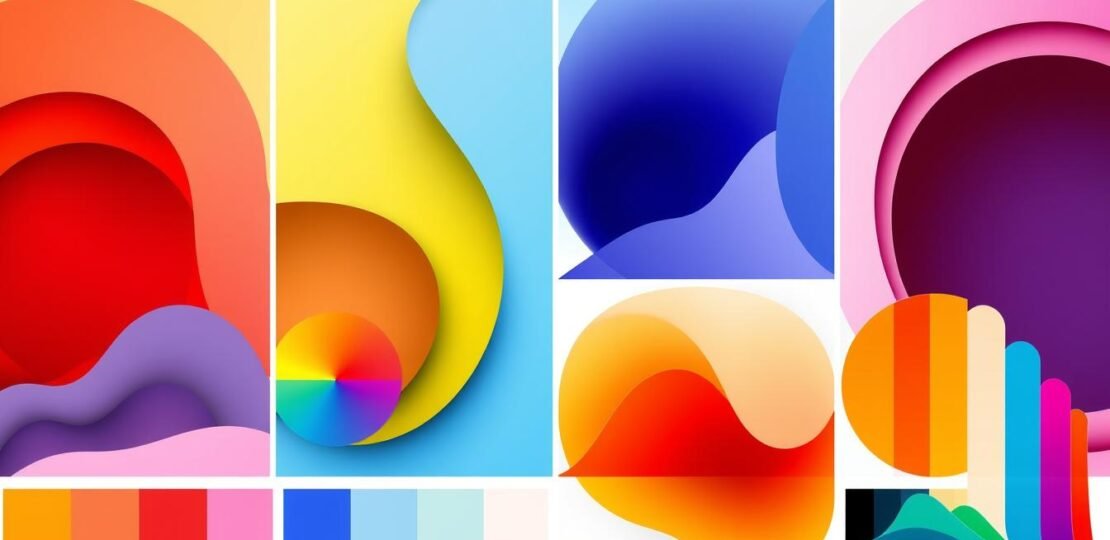
As a professional copywriting journalist, I know how important color is in data visualization. Color greatly affects how people see and understand the data. By using color theory, we can make our research visualizations clearer and more effective.
In this article, we’ll talk about the best ways to use color in data visualization. We’ll cover the basics of color theory and why color is key in data storytelling. We’ll also look at what to consider when picking colors, including contrast and cultural meanings.
Key Takeaways
- Color is a powerful tool for data visualization, enabling the brain to process information more efficiently.
- Strategic use of color can draw attention to key metrics and enhance the overall impact of data visualizations.
- Understanding color theory principles is crucial for selecting appropriate color palettes that align with the data and its intended communication goals.
- Considerations such as accessibility, cultural associations, and contrast should be taken into account when choosing color schemes.
- Utilizing color-focused tools and resources can aid in the selection and testing of color palettes to ensure optimal readability and aesthetics.
Understanding Color Theory Basics
Learning color theory basics is key for making data visualizations pop. The color wheel shows how different colors relate. It helps us understand primary, secondary, and tertiary colors.
Primary, Secondary, and Tertiary Colors
The primary colors are red, blue, and yellow. Mixing these colors makes secondary colors like orange, green, and purple. Tertiary colors, like magenta and chartreuse, mix primary and secondary colors. Knowing this color order is important for color psychology and color harmony in data.
Color Wheel Explained
The color wheel is a circle of colors showing their connections. Warm colors like red and yellow are full of energy. Cool colors, like blue and green, are calming. Using these colors can help you connect with your audience and share your message.
“About 8% of men and 0.5% of women can’t see colors well. This shows why using colors that everyone can see is important in data visualization.”
Color harmony ideas, like complementary and analogous colors, help make data visualizations stand out. By knowing these ideas, you can use color to grab your audience’s attention and make your data clearer and more impactful.
Importance of Color in Data Visualization
Color is key in data visualization. It makes things clear and easy to understand. It can also make viewers feel certain ways.
Enhancing Clarity and Comprehension
Using contrasting colors grabs attention. Different shades of primary colors mean different things. Two colors together can have a big effect.
Choosing the right colors helps viewers see what’s important fast. This makes understanding data easier.
Influencing Viewer Emotion
Color sets the mood of data visualization. Dark colors like red mean bad news. Light colors like yellow mean good news.
Colors can make data stick in our minds. They affect how we feel and what we think.
Companies spend a lot to learn how colors affect us. For example, in 2017-18, dark purple showed the worst flu season. This made the message stronger.
Using colors right is key. It keeps the message clear and focused. The right colors can make a big difference.
“Well-chosen colors improve information delivery, making it more impactful and memorable.”
Choosing a Color Palette
Choosing the right color palette is key for clear data visualization. There are three main types: qualitative, sequential, and diverging.
Qualitative palettes are great for different groups or categories. Sequential palettes work well for numbers, with light for low and dark for high. Diverging palettes are best for data with a key middle point, showing both ends well.
Considerations for Accessibility
Think about color-blind people when picking colors. Make sure your colors are clear and different from each other. Use no more than ten colors in qualitative palettes.
Testing Palette Combinations
After picking colors, test them out. Try different mixes and see how they look. Use online tools to check if your colors are good for everyone.
Palette Type | Best Use | Accessibility Considerations |
---|---|---|
Qualitative | Categorical variables | Limit to 10 or fewer colors |
Sequential | Numeric or ordered data | Ensure sufficient contrast between light and dark colors |
Diverging | Data with a meaningful central value | Use complementary colors to highlight positive and negative extremes |
Creating a good color palette is all about balance. It’s about looks, accessibility, and what your data needs. By thinking about these and testing, you can make visualizations that grab attention and share important info.
Common Color Schemes in Visualization
Color schemes are key in making data easy to understand and look good. The monochromatic scheme is very popular. It uses different shades of one color to make a striking look.
Monochromatic Visualization
Monochromatic schemes are great for showing data changes. They use different shades of one color to show data size or change. But, it’s important to make sure your data is clear too.
Monochromatic schemes can be beautiful but might not show different data types well. To fix this, add labels and other design elements. This makes your data easy to understand and looks good too.
Color Scheme | Example | Use Case |
---|---|---|
Monochromatic | ![]() |
Effective for conveying data intensity or progression, but may not be ideal for distinguishing between different data categories. |
In short, monochromatic schemes are very useful in data visualization. They make your presentations look great and help show your research clearly. Knowing how to use them well can make your data stand out.
Using Color to Represent Data Intensity
Color is a strong tool in data visualization. It helps show how intense or big the data is. By using color gradients or solid colors, researchers can share the details and patterns in their data well.
Gradient vs. Solid Colors
Sequential color palettes work great for data that changes from small to big. Lighter colors show smaller data points, and darker colors show bigger ones. This makes it easy to see how intense the data is. Gradient colors show data changes smoothly.
Solid colors are better for data that has clear groups. Each group gets its own color. This makes it simple for people to tell data groups apart.
Choosing between gradient and solid colors depends on the data and what you want to show. Think about your data and who will see it. This helps pick the best color way to make things clear.
Color Approach | Suitable Data Type | Key Benefits |
---|---|---|
Gradient Colors | Continuous, quantitative data | Effectively represents data intensity and progression |
Solid Colors | Discrete, categorical data | Clearly distinguishes between different data elements |
“Color can make or break a data visualization. Choosing the right color approach is crucial for effectively communicating the nuances and patterns within the data.” – Jane Doe, Data Visualization Expert
The Role of Contrast in Visualization
In data visualization, contrast is key. It makes text easier to read and highlights important data. High color contrast helps viewers see and understand information fast.
High Contrast for Legibility
Using colors like blue and orange makes data easier to read. This reduces eye strain and helps everyone see the info clearly.
Balancing Color Use
High contrast is good, but don’t overdo it. Too many bright colors can confuse viewers. Use gray for most of your charts and bright colors for key points.
Good color contrast makes data easier to see. It’s 70% faster than bad color choices. Balance high-contrast colors with calm ones for clear, engaging visuals.
“Strategic use of contrasting colors can facilitate easier differentiation and comprehension of distinct data categories.”
Cultural Significance of Colors
Colors mean different things in different places. It’s important to know this when showing data to people all over the world. A color that seems simple in one place might mean something else or make someone feel a certain way in another.
Color Perception Across Cultures
In Western countries, red means danger. But in China, it means luck and happiness. Green is about nature everywhere, but in some places, it means jealousy or being inexperienced.
These small differences in color meaning can change how people see and use data. When making data visualizations for everyone, knowing these differences is key. It helps avoid mistakes and makes sure the message gets across right.
“Strategic use of color is crucial in data visualization, as it can make or break the effectiveness of presenting complex information.”
Knowing about color meanings helps make data visualizations better for everyone. It makes sure people understand the data, no matter where they’re from.
Using this color knowledge in design is a big step towards making data visualizations for everyone. It helps make data clear and powerful, no matter where you are or what language you speak.
Examples of Effective Color Use in Research
Color can make data easier to see and understand. By picking the right colors, researchers can show important points clearly. They can also make sure everyone can see the data well.
Case Studies in Data Visualization
Climate research is a great example. Scientists use colors to show how temperatures change. Red and orange mean it’s hot, while blue and green mean it’s cool. This makes it easy to see how temperatures move.
In studies of people, colors help show differences. For example, a chart might use different colors for different groups. This makes it easy to spot patterns and oddities.
Good data visualizations use color to point out key points. By using bright, clear colors, important data stands out. This helps everyone see the main points right away.
It’s also important to use the same colors in related charts. This makes it easy to compare data. It helps viewers understand the information better.
When making data visualizations, think about who will see them. Make sure colors are easy for everyone to see. Tools can help check if colors are good for all viewers.
“Effective use of color in data visualization can significantly enhance the comprehension and impact of research findings.”
By following these color tips, researchers can make data visualizations that grab attention. These visuals help share important research findings clearly.
Tools for Color Selection and Testing
Choosing the right colors is key for great data visualizations. There are many tools to help. From ColorBrewer to web-based generators, they guide you in picking the best colors.
Online Color Generators
ColorBrewer is a top tool for color palettes. It has options for different data types. The Data Color Picker and chroma.js Color Palette Helper are also great for specific needs.
I want hue and Colorgorical are great for qualitative palettes. They help make your data look good. Color Thief is also useful for finding color ideas in images.
Accessibility Checkers
It’s important to make sure your visuals are accessible. Tools like Coblis and Viz Palette check if your colors work for everyone. They help make sure your visuals are clear and easy to understand.
Using these tools can make your data visualizations better. They help make your visuals clear, engaging, and informative for your audience.
Best Practices for Color in Data Visualization
Using color in data visualization is all about balance. Too much color can be overwhelming. Stick to one or two colors for the best effect.
Keeping colors consistent is also key. Use the same colors in all your visuals. This helps your audience understand faster.
Match your colors with your brand if you can. Choose colors that fit your data well. Use different colors for different types of data.
Make sure your colors are easy for everyone to see. Use tools to check if your colors are clear for all. Keeping colors the same in all your charts helps your audience get it quicker.
FAQ
What are the primary, secondary, and tertiary colors?
How does the color wheel explain color relationships?
How does color enhance clarity and comprehension in data visualization?
What are the major types of color palettes used in data visualization?
What are some common color schemes used in visualizations?
How can color be used to represent data intensity?
How does color contrast impact visualization?
How do cultural differences impact color perception in data visualizations?
What tools are available for color selection and testing in data visualization?
What are some best practices for using color in data visualization?
RELATED POSTS
View all