The Best AI Data Analysis Tools for Researchers in 2024
November 16, 2024 | by Jean Twizeyimana

The world of research is undergoing a transformative shift, with AI-powered data analysis tools taking center stage. These innovative solutions are revolutionizing the way researchers explore, interpret, and derive insights from vast datasets, accelerating discovery and enhancing accuracy across diverse fields. As we move into the year 2024, the landscape of AI Data Analysis Tools, Data Mining Software, and Predictive Analytics Solutions is poised to reach new heights, offering researchers unparalleled capabilities to unlock the full potential of their research endeavors.
Key Takeaways
- AI-powered data analysis tools are revolutionizing research by automating data processing, enhancing literature reviews, and generating actionable insights.
- The top AI data analysis tools for 2024 include RapidMiner, Tableau, Microsoft Azure Machine Learning, KNIME, and Google Cloud AutoML, among others.
- These tools offer a range of features, from user-friendly interfaces to advanced analytical capabilities, catering to the diverse needs of researchers.
- Researchers can leverage free and paid AI data analysis tools, depending on their specific requirements and budget considerations.
- AI is transforming data analysis by enhancing predictive analytics, automating data cleaning, and enabling real-time processing, driving breakthroughs in various industries.
Introduction to AI Data Analysis Tools
The world of research is being transformed by the advent of AI data analysis tools. These innovative technologies leverage the power of artificial intelligence and machine learning to assist researchers in navigating the complexities of data-driven investigations. From automating data collection and preparation to generating actionable insights, AI data analysis tools are becoming indispensable for researchers across various disciplines.
What Are AI Data Analysis Tools?
AI data analysis tools are software applications that employ advanced algorithms and machine learning models to analyze large, complex datasets. These tools can streamline tasks such as data cleaning, data visualization, and statistical analysis, allowing researchers to uncover hidden patterns and trends with greater speed and accuracy.
Importance for Researchers
For researchers, AI data analysis tools are invaluable assets. They can boost productivity, improve the reliability of findings, and facilitate interdisciplinary collaboration. By automating repetitive tasks and providing intelligent insights, these tools free up researchers to focus on the strategic aspects of their work, ultimately driving innovation and transforming the way research is conducted.
Overview of Current Trends
The adoption of AI data analysis tools is on the rise, with a growing emphasis on improving efficiency, precision, and decision-making. Machine Learning Platforms and Natural Language Processing (NLP) Tools are at the forefront of this trend, enabling researchers to extract insights from vast datasets and unstructured information sources with unprecedented ease and accuracy.
As the demand for data-driven research continues to grow, the landscape of AI data analysis tools is rapidly evolving, offering researchers an ever-expanding array of options to streamline their workflows and unlock new possibilities in their respective fields.
Key Features to Look For
As researchers explore the transformative potential of AI-driven data analysis, it’s crucial to consider the key features that set the best tools apart. From user-friendly interfaces to advanced analytical capabilities, the right AI data analysis software can streamline workflows, enhance predictive insights, and drive more informed decision-making.
User-Friendly Interfaces
Intuitive and visually engaging user interfaces are essential for driving widespread adoption and productivity. Leading Text Analytics Software and Sentiment Analysis Tools prioritize simplicity, allowing researchers to easily navigate data exploration, visualization, and model-building tasks without a steep learning curve.
Advanced Analytical Capabilities
Beyond basic reporting and data visualization, the most powerful AI data analysis tools offer a suite of advanced analytical features. This includes robust predictive analytics capabilities, automated data cleaning and preparation processes, and real-time data processing for timely insights.
Integration with Other Software
Seamless integration with existing research software, databases, and other data sources is crucial for streamlining workflows and maximizing productivity. Look for AI data analysis tools that offer seamless connectivity, allowing researchers to leverage their existing technology investments and avoid data silos.
Key Feature | Importance for Researchers |
---|---|
User-Friendly Interfaces | Enhance adoption and productivity by simplifying complex data analysis tasks |
Advanced Analytical Capabilities | Unlock predictive insights, automate data preparation, and enable real-time processing |
Integration with Other Software | Streamline workflows and leverage existing technology investments |
Top AI Data Analysis Tools of 2024
As researchers navigate the ever-growing landscape of data, the demand for powerful, AI-driven data analysis tools has never been higher. In 2024, several innovative solutions are leading the charge, offering researchers advanced capabilities in Data Visualization Dashboards and Business Intelligence (BI) Solutions.
Scite: Evaluating Citation Quality
Scite is a cutting-edge tool that utilizes machine learning algorithms to evaluate the quality and context of academic citations. By analyzing the way a research paper has been cited, Scite provides valuable insights into the significance and impact of the work, empowering researchers to make more informed decisions.
Consensus: Synthesizing Research Findings
Consensus takes a unique approach to data analysis by leveraging natural language processing (NLP) to synthesize and summarize research findings from multiple sources. This AI-powered tool helps researchers quickly identify key trends, patterns, and consensus within the existing body of literature, streamlining the research process.
Connected Papers: Visualizing Research Relationships
Connected Papers is a visually striking tool that employs co-citation analysis to map the relationships between academic papers. By generating interactive visualizations, this AI-driven solution allows researchers to explore the interconnected web of scholarly work, fostering new insights and potential collaborations.
RapidMiner: Intuitive Data Analysis
RapidMiner offers an intuitive, user-friendly interface that caters to researchers of all skill levels. With its drag-and-drop framework and advanced analytical capabilities, RapidMiner empowers users to quickly and effectively harness the power of AI for their data analysis needs, from predictive modeling to automated data processing.
These cutting-edge AI data analysis tools, each with its unique strengths, are poised to transform the way researchers approach their work in 2024 and beyond. By leveraging the latest advancements in machine learning, natural language processing, and data visualization, these solutions are redefining the boundaries of what is possible in the realm of data-driven research and discovery.
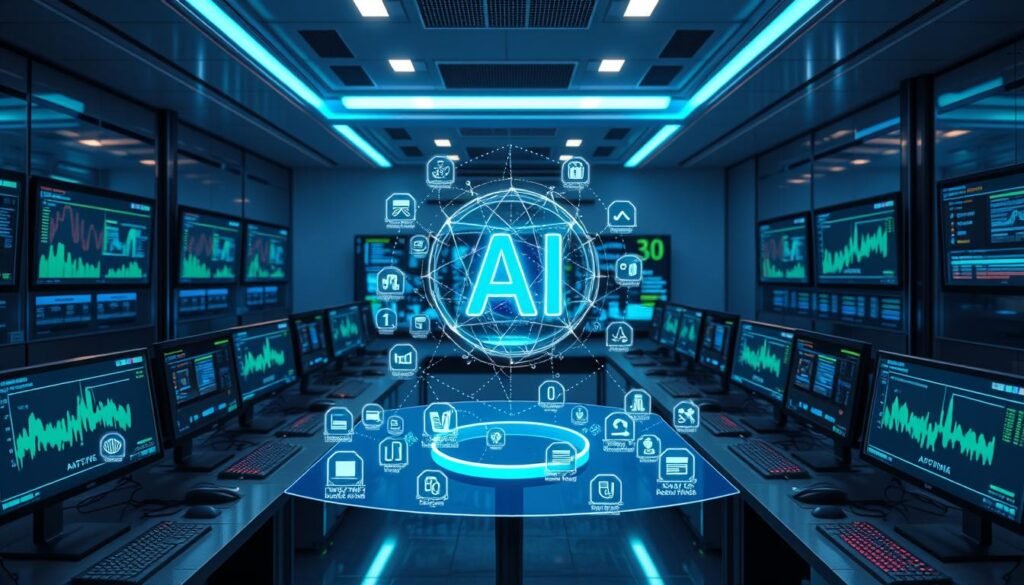
Comparison of Free vs. Paid Tools
When it comes to leveraging Big Data Analytics Platforms and AI Data Analysis Tools, researchers have the option to choose between free and paid solutions. Each approach offers unique benefits, and understanding the tradeoffs is crucial in selecting the right tool for your research needs.
Benefits of Free AI Tools
Free AI tools often provide basic functionalities and are suitable for small-scale projects or individual researchers. These tools offer an opportunity to explore AI capabilities without financial commitment. For instance, the Harvard AI Sandbox provides access to 25% of AI tools for general use, all classified as Level 3 data and below, allowing researchers to experiment and familiarize themselves with the technology.
Advantages of Paid AI Data Analysis Tools
Paid AI Data Analysis Tools typically offer more advanced features, better support, and enhanced security. They are often more suitable for large-scale research projects or organizations. Currently, there are 8 AI developer tools available for integration of Large Language Models (LLMs), all of which require a request from Harvard University Information Technology (HUIT) for access. Additionally, 75% of AI tools for general use require coordination through Schools and Units for access, underscoring the need for institutional support for paid solutions.
Cost-Benefit Analysis
When conducting a cost-benefit analysis, researchers should consider factors such as the scale of their research, the required features, data security needs, and their available budget. For example, RapidMiner offers an array of classification, regression, clustering, and association rule mining algorithms, while Orange is praised for its user-friendly, color-coordinated interface. On the other hand, free tools like KNIME and Datawrapper can provide valuable data cleaning and visualization capabilities for specific needs.
Feature | Free AI Tools | Paid AI Tools |
---|---|---|
Advanced Analytical Capabilities | Limited | Comprehensive |
Data Security and Privacy | Basic | Enhanced |
Technical Support | Limited | Dedicated |
Scalability | Suitable for small-scale projects | Suitable for large-scale research |
By carefully evaluating the benefits and limitations of both free and paid AI Data Analysis Tools, researchers can make an informed decision that aligns with their project requirements and budget constraints, ultimately enhancing their data analysis capabilities and research outcomes.
How AI Transforms Data Analysis
The world of data analysis is undergoing a profound transformation, thanks to the integration of Artificial Intelligence (AI) and Machine Learning (ML) technologies. These advanced tools are revolutionizing the way researchers and data analysts approach their work, enhancing predictive analytics, automating data cleaning processes, and enabling real-time data processing.
Enhanced Predictive Analytics
AI-powered Predictive Analytics Solutions are empowering researchers to forecast trends and outcomes with unprecedented accuracy. By analyzing historical data and identifying patterns, these tools can provide invaluable insights to support data-driven decision-making and strategic planning. This is particularly beneficial in industries such as healthcare, finance, and logistics, where the ability to anticipate future events can have a significant impact.
Automated Data Cleaning Processes
One of the most time-consuming and tedious tasks in data analysis is data cleaning, but AI is changing that. Machine Learning Platforms can automate this process, significantly reducing manual effort and improving data quality. By identifying and addressing inconsistencies, missing values, and other data anomalies, these AI-driven tools ensure that researchers have access to clean, reliable data, enabling them to focus on higher-level analysis and interpretation.
Real-Time Data Processing
The speed at which data is generated and the need for continuous monitoring have created a demand for real-time data processing capabilities. AI-powered analytics solutions can process and analyze data in real-time, keeping researchers updated on the latest developments and trends. This allows for more agile decision-making and the ability to respond to changing conditions swiftly, particularly in fast-paced industries or during dynamic events.
The integration of AI in data analysis has transformed the field, empowering researchers to uncover deeper insights, automate tedious tasks, and stay ahead of the curve. As AI continues to advance, the future of data analysis looks increasingly promising, with the potential to unlock new levels of understanding and innovation.
Industry-Specific Applications
As the field of Natural Language Processing (NLP) and Text Analytics Software continues to evolve, the applications of AI data analysis tools have expanded across various industries. These versatile tools are empowering researchers, businesses, and organizations to derive actionable insights from complex datasets, streamlining decision-making processes and driving innovation.
Healthcare and Medical Research
In the healthcare and medical research sectors, AI data analysis tools are proving invaluable. These tools assist in analyzing vast troves of medical data, identifying patterns in diseases, and accelerating drug discovery processes. For example, IBM Watson Analytics has been utilized in healthcare to accurately analyze X-rays and CT scans for diseases like pneumonia and tuberculosis, detect lung nodules, and identify osteoporosis in X-rays. AI-powered analytics also enable personalized treatment plans through the analysis of genomic data, patient information integration, and predictive analytics.
Social Sciences and Behavioral Studies
Within the realm of social sciences and behavioral studies, AI data analysis tools are transforming the way researchers approach large-scale data processing and analysis. Tools like AnswerRocket, a search-powered AI data analytics platform, allow for data retrieval and analysis through natural language queries, facilitating the interpretation of complex survey data and social media trends. These AI-driven insights can help identify behavioral patterns and inform evidence-based decision-making in fields such as psychology, sociology, and anthropology.
Marketing and Business Analytics
In the marketing and business analytics sphere, AI-powered tools are revolutionizing the way organizations derive valuable insights from data. Solutions like Qlik Sense, which unifies data sources and develops visual analytics within a secure governance framework, empower both data engineers and business users to collaborate effectively. AI-driven sentiment analysis, customer behavior prediction, and market trend forecasting enabled by tools like Google Cloud AutoML are transforming the way businesses make strategic decisions and optimize their marketing campaigns.
Industry | AI Data Analysis Applications | Example Tools |
---|---|---|
Healthcare and Medical Research |
| IBM Watson Analytics, Polymer |
Social Sciences and Behavioral Studies |
| AnswerRocket, Bardeen |
Marketing and Business Analytics |
| Qlik Sense, Google Cloud AutoML |
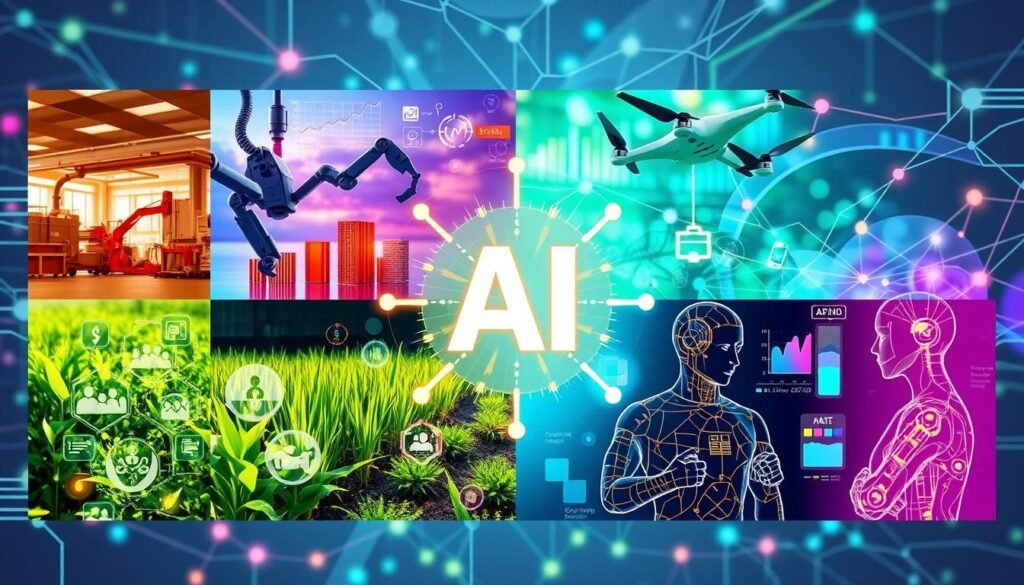
Best Practices for Using AI Tools
As researchers delve into the world of data analysis, leveraging the power of AI tools is becoming increasingly vital. However, to truly harness the benefits of these advanced technologies, it’s essential to follow best practices that ensure accurate and insightful results. From Data Visualization Dashboards to sophisticated Business Intelligence (BI) Solutions, AI-powered tools offer a wealth of opportunities for researchers to uncover hidden patterns and make data-driven decisions.
Data Preparation Techniques
Thorough data preparation is the foundation for successful AI-powered analysis. This involves meticulous cleaning, structuring, and normalizing of data to ensure it is ready for processing by AI algorithms. By taking the time to curate and refine the dataset, researchers can minimize the risk of inaccurate findings and enhance the reliability of their insights.
Effective Visualization Strategies
Presenting complex data in a clear and intuitive manner is crucial for communicating findings to stakeholders. AI-powered visualization tools, such as Tableau, excel at creating dynamic dashboards and interactive data visualizations that make it easier to interpret and share insights. By leveraging these strategies, researchers can ensure their AI-driven analyses are easily understood and actionable.
Interpreting AI Findings
When it comes to interpreting the results of AI-driven analyses, researchers must approach with a critical eye. It’s essential to validate the findings against domain knowledge and conduct statistical tests to ensure the accuracy and reliability of the conclusions. Additionally, researchers should be mindful of potential biases in AI models and take steps to mitigate them. Regular monitoring and updating of AI models is also crucial to account for changing data patterns and business requirements.
By following these best practices, researchers can leverage the power of AI-driven data analysis tools to unlock valuable insights, enhance decision-making, and drive meaningful progress in their respective fields. As the field of data analysis continues to evolve, mastering the art of effective AI tool usage will be a key differentiator for researchers seeking to stay ahead of the curve.
Case Studies: Success Stories
The transformative power of AI Data Analysis Tools is evident in the success stories spanning various sectors. In university research projects, tools like Scite and Connected Papers have revolutionized literature reviews and citation analysis, significantly reducing research time and improving the quality of academic outputs.
In the realm of corporate data analytics, companies like Google and NASA have leveraged Data Mining Software such as Elicit to synthesize top research papers and automate tedious research tasks. This has led to enhanced efficiency and productivity, ultimately driving innovation and business growth.
Non-profit organizations have also embraced the power of AI tools for impact assessments. By analyzing large datasets, these organizations can measure the effectiveness of their programs and make data-driven decisions for resource allocation, leading to greater social impact.
- University Research Projects: Scite and Connected Papers have revolutionized literature reviews and citation analysis, reducing research time and improving academic output quality.
- Corporate Data Analytics: Google and NASA utilize tools like Elicit to synthesize research papers and automate tasks, boosting efficiency and driving innovation.
- Non-Profit Impact Assessments: AI tools enable organizations to analyze data, measure program effectiveness, and make data-driven decisions for greater social impact.
These success stories demonstrate the transformative potential of AI Data Analysis Tools and Data Mining Software in various industries, from academia to corporate and non-profit settings. By embracing these cutting-edge technologies, organizations can unlock new levels of productivity, innovation, and social impact.
Common Challenges in AI Data Analysis
As researchers and organizations increasingly leverage Sentiment Analysis Tools and Big Data Analytics Platforms to unlock valuable insights, they face several common challenges in AI data analysis. These obstacles range from concerns over data privacy to managing data quality and addressing bias in AI models.
Data Privacy Concerns
One of the primary challenges in AI data analysis is ensuring the privacy and security of sensitive information. When dealing with personal or confidential data, researchers must navigate a complex web of regulations and ethical considerations to protect individuals’ rights. Failure to do so can result in costly data breaches and reputational damage.
Managing Data Quality
The effectiveness of AI tools heavily depends on the quality of the input data. Inaccurate, incomplete, or biased data can lead to flawed analyses and unreliable results. Maintaining high-quality data requires robust data management practices, including regular audits, cleaning, and validation processes.
Dealing with Bias in AI Models
AI models can inadvertently introduce biases due to the data they are trained on, which may reflect historical biases or societal prejudices. Addressing these biases is crucial to ensure fair and unbiased decision-making. Researchers must carefully examine their AI models for potential sources of bias and implement strategies to mitigate them.
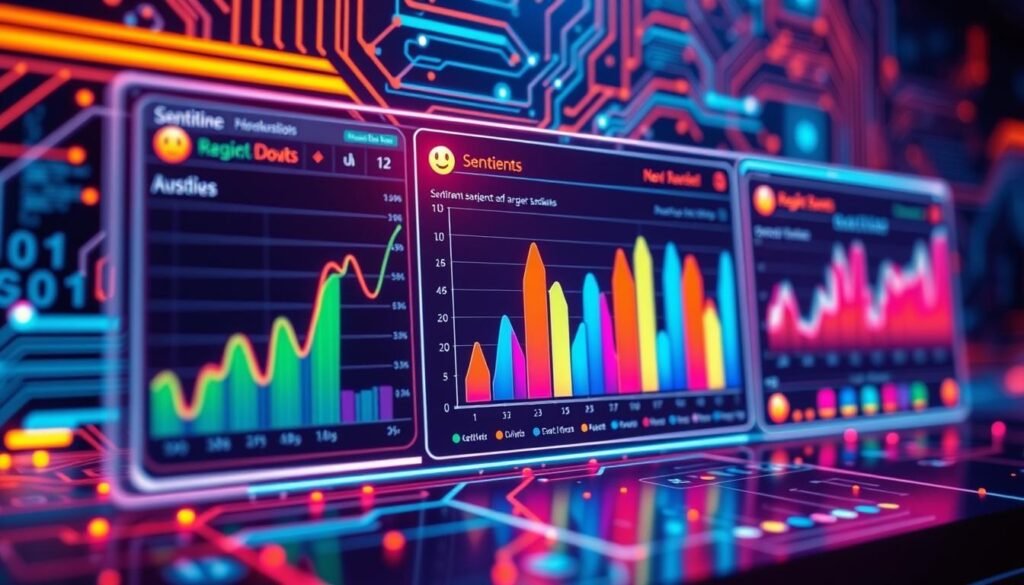
Other challenges in AI data analysis include the potential for AI hallucination, where systems generate incorrect or nonsensical information, and the lack of human insight in interpreting nuanced data. Overcoming these hurdles requires a multifaceted approach, including robust data governance, interdisciplinary collaboration, and continuous model evaluation and refinement.
Future Trends in AI Data Analysis
As the world of data analysis continues to evolve, researchers and professionals can expect to see some exciting advancements in the field of artificial intelligence (AI). One of the key trends is the increased automation in research processes, with AI tools taking on more complex tasks and freeing up researchers to focus on high-level analysis and interpretation.
Another emerging trend is the integration of AI with big data technologies. As the volume and complexity of data continue to grow, the seamless integration of AI and big data solutions will become crucial, allowing for more comprehensive and nuanced analyses. This integration will enable researchers to unlock valuable insights that were previously hidden within massive datasets.
Alongside these technological advancements, the ethical considerations in AI use will also come into sharper focus. Researchers and organizations will need to prioritize the development of fair, transparent, and accountable AI systems that respect privacy, mitigate bias, and adhere to ethical principles.
Additionally, there is a trend towards more user-friendly AI tools that don’t require extensive coding knowledge. This democratization of advanced analytics will make these powerful capabilities more accessible to a wider range of researchers and professionals, empowering them to harness the full potential of Machine Learning Platforms and Natural Language Processing (NLP) Tools in their work.
As the field of AI data analysis continues to evolve, researchers can look forward to a future where their work is enhanced by intelligent, automated systems that provide deeper insights, faster processing, and more ethical decision-making. By staying attuned to these trends, researchers can position themselves to take full advantage of the latest advancements in this rapidly-changing landscape.
User Testimonials: Real-Life Experiences
The true power of AI data analysis tools is best seen through the real-life experiences of those who use them daily. Researchers, data analysts, and IT professionals across various industries have praised the transformative impact of these innovative solutions.
Feedback from Researchers
Researchers have reported significant time savings and improved accuracy in their literature reviews by utilizing AI-powered tools like Scite and Consensus. These applications harness the power of Text Analytics Software to quickly identify relevant research articles, extract key insights, and streamline the knowledge synthesis process.
Insights from Data Analysts
Data analysts have highlighted the predictive capabilities of platforms like RapidMiner and DataRobot, noting enhanced decision-making processes and more accurate forecasting. These Data Visualization Dashboards empower analysts to uncover hidden patterns, trends, and correlations within complex datasets, leading to more informed and strategic business decisions.
Perspectives from IT Professionals
IT professionals have praised the scalability and seamless integration capabilities of cloud-based AI data analysis solutions, such as Microsoft Azure Machine Learning and Google Cloud AutoML. These enterprise-level platforms allow organizations to effortlessly deploy and scale AI-driven insights across their operations, driving operational efficiencies and enhanced problem-solving capabilities.
These real-life experiences from diverse professional backgrounds underscore the transformative impact of AI data analysis tools. As researchers, analysts, and IT experts continue to harness the power of these innovative solutions, the future of data-driven decision-making looks brighter than ever.
Conclusion: Choosing the Right Tool
Selecting the most suitable AI data analysis tool for research requires careful consideration of your specific objectives, data types, analytical needs, and available resources. User-friendly interfaces, advanced analytical capabilities, seamless integration with existing systems, and cost-effectiveness are key factors to evaluate when making your choice.
Recap of Key Points
Throughout this article, we’ve explored a diverse range of AI data analysis tools, from popular options like Microsoft Excel and Google Data Studio to more specialized solutions like Python, R, and Apache Hadoop. Each tool offers unique strengths, catering to different research requirements and data analysis needs. It’s essential to understand the specific capabilities of these tools and how they align with your research goals.
Recommendations for Researchers
For researchers, it’s advisable to start with free or trial versions of AI data analysis tools to gain hands-on experience and understand their capabilities. This approach allows you to assess the user-friendliness, analytical depth, and integration potential before investing in paid solutions. As your research evolves, consider upgrading to more advanced tools that offer features tailored to your specific requirements, such as predictive analytics, automated data cleaning, or real-time data processing.
Final Thoughts on the Future of AI Data Analysis Tools
As the field of artificial intelligence continues to advance, the capabilities of data analysis tools will become increasingly sophisticated. Researchers can expect to see more automated, accurate, and insightful data analysis solutions that leverage the power of AI. However, it’s crucial to maintain a balance between leveraging these technological advancements and applying human expertise and ethical considerations throughout the research process. By carefully selecting the right AI data analysis tools and using them judiciously, researchers can unlock new possibilities in their fields of study and drive meaningful discoveries.
Related Articles
- Exploring The AI Qualitative Data Analysis in Surveys Now
- Revolutionize Your Research: Machine Learning Survey Analysis in 2024
- Latest AI in Survey Research: From Design to Analysis
- The Ultimate Guide to AI in Survey Research
- The Latest AI Sentiment Analysis Techniques for Survey Responses
- How To Overcome Survey Data Bias Using AI
- How To Leverage Natural Language Processing (NLP) for Open-Ended Survey Questions
FAQ
What are AI data analysis tools?
Why are AI data analysis tools important for researchers?
What are the current trends in the adoption of AI data analysis tools?
What key features should I look for when selecting an AI data analysis tool?
What are some of the top AI data analysis tools for 2024?
What are the advantages and disadvantages of free vs. paid AI data analysis tools?
How does AI transform data analysis in research?
What are some industry-specific applications of AI data analysis tools?
What are the best practices for using AI data analysis tools?
Can you provide examples of successful case studies using AI data analysis tools?
What are the common challenges in using AI data analysis tools?
What are the future trends in AI data analysis?
What do user testimonials say about the practical applications of AI data analysis tools?
Source Links
- Top 10 Must Use AI Tools for Data Analysis [2024 Edition]
- Top 10 AI Analytics Tools for Data Analysis in 2024
- AI data analytics
- How to Use ChatGPT’s Advanced Data Analysis Feature – MIT Sloan Teaching & Learning Technologies
- Top 7 AI Tools for Data Analysis: Ultimate Guide
- Using AI for Data Analysis: The Ultimate Guide (2024) | Luzmo
- Best AI for Data Analysis: Our Top Picks
- 17 Generative AI Data Analytics Tools Everyone Should Know About
- Top 12 AI Tools for Data Analysis To Include In Your Tech Stack
- Comparison of Generative AI Tools at Harvard
- 10 Data Analysis Tools and When to Use Them
- AI in Data Analysis: How Artificial Inteligence Enhances Analytics?
- How AI Is Rewriting the Rules of Data Analysis | Analyst Catalyst Blog
- Top 6 AI Tools for Data Analytics
- AI Use Cases & Applications Across Major industries
- How To Use AI For Data Analysis: A Step-By-Step Guide
- How to Use AI in Data Analysis? The Complete Guide
- 7 Ways to Use AI For Data Analytics [Examples & Tools] | Airbyte
- AI in Action: 6 Business Case Studies on How AI-Based Development is Driving Innovation Across Industries
- 185 real-world gen AI use cases from the world’s leading organizations
- Data Analytics Case Studies: Success Stories from Various Industries
- 7 Common Data Analytics Problems – & How to Solve Them
- 9 Common Challenges to AI Adoption and How to Avoid Them
- AI in Data Analytics: Transforming Insights and Decision-Making
- Five Key Trends in AI and Data Science for 2024 | Thomas H. Davenport and Randy Bean
- AI Success Stories
- 10 Real-World Examples of AI-Powered Sentiment Analysis
- Build Generative AI Applications with Foundation Models – Amazon Bedrock Customer Testimonials – AWS
- How to Choose the Perfect Data Analysis Tool: A Step-by-Step Guide
- AI Tools for Data Analysis: The Ultimate Guide [Updated]
RELATED POSTS
View all