Predictive Analytics in Research: How AI Can Improve Forecasting Now
November 15, 2024 | by Jean Twizeyimana

AI in Forecasting? In today’s data-driven world, the field of predictive analytics has emerged as a powerful tool for researchers and businesses alike. At the heart of this revolution is the integration of Artificial Intelligence (AI) and machine learning. AI-powered predictive analytics harnesses the power of historical data, sophisticated algorithms, and computational processing to forecast future trends with unparalleled accuracy and speed.
Machine learning algorithms in AI predictive analytics learn from data over time to make predictions based on historical patterns and relationships. This advanced technique goes beyond traditional forecasting methods, which often struggle to keep pace with the complexity and volume of data in today’s markets. By leveraging AI, researchers can uncover deep insights, identify emerging opportunities, and mitigate potential risks in real-time, empowering them to make more informed and data-driven decisions.
At the core of AI-driven predictive analytics are three key components: Data, Algorithms, and Predictions. By collecting and preparing relevant data, applying advanced machine learning models, and generating actionable insights, researchers can transform raw information into a powerful tool for strategic planning and decision-making.
Key Takeaways
- AI-powered predictive analytics harnesses historical data and sophisticated algorithms to forecast future trends with unparalleled accuracy.
- Machine learning algorithms in AI predictive analytics learn from data over time to make data-driven predictions.
- AI-based predictive analytics leverages three core components: Data, Algorithms, and Predictions to transform raw data into actionable intelligence.
- The integration of AI into predictive analytics enables researchers to uncover deep insights, identify emerging opportunities, and mitigate potential risks in real-time.
- AI-powered predictive analytics empowers researchers to make more informed and data-driven decisions, leading to enhanced strategic planning and decision-making.
Understanding AI in Forecasting
Artificial Intelligence (AI) has emerged as a transformative force in the realm of forecasting, revolutionizing the way organizations analyze data and make predictions. AI-driven forecasting utilizes sophisticated Natural Language Processing, Deep Learning Algorithms, and advanced Data Mining Techniques to process information from diverse sources, identify hidden trends, and generate predictions that exceed human capabilities.
Definition of AI in Forecasting
AI in forecasting involves the application of machine learning algorithms and statistical models to analyze historical data, identify patterns, and generate accurate predictions about future events or trends. This process goes beyond traditional forecasting methods, such as time series analysis, moving averages, and exponential smoothing, by uncovering complex relationships and non-linear patterns within the data.
Importance in Predictive Analytics
The integration of AI into the forecasting process has significantly enhanced the field of predictive analytics. By leveraging AI’s ability to handle large, diverse datasets and identify intricate patterns, organizations can now make more accurate, data-driven decisions. AI-powered forecasting models can be configured for real-time predictions, enabling businesses to respond quickly to changing market conditions and consumer preferences.
Furthermore, AI forecasting can mitigate human bias in predictions by providing objective, data-driven insights. This not only improves the reliability of forecasts but also empowers organizations to make strategic decisions with greater confidence.
Key Benefits of AI for Forecasting
Artificial Intelligence (AI) has revolutionized the world of forecasting, offering a suite of powerful capabilities that have transformed decision-making across various industries. By leveraging advanced Forecasting Accuracy, Data Analysis, and Predictive Modeling techniques, AI-driven forecasting solutions are delivering unprecedented levels of accuracy, speed, and flexibility.
Increased Accuracy and Reliability
AI-powered forecasting models excel at identifying complex patterns and relationships within massive datasets, allowing them to generate more accurate and reliable predictions. These models can process vast amounts of historical data, external market factors, and real-time inputs to uncover insights that traditional forecasting methods often miss.
Enhanced Speed of Data Processing
Compared to manual data analysis, AI can process information at lightning-fast speeds, enabling organizations to make timely, data-driven decisions. AI algorithms can rapidly sift through structured and unstructured data, automate repetitive tasks, and deliver insights in real-time, significantly improving the efficiency of the forecasting process.
Ability to Handle Complex Data
AI-based forecasting solutions are equipped to handle the increasing complexity of data sources, including social media, IoT sensors, and evolving customer behavior. By leveraging advanced Predictive Modeling techniques, these systems can extract valuable insights from diverse and high-dimensional data, leading to more comprehensive and nuanced forecasts.
Benefit | Description |
---|---|
Increased Accuracy and Reliability | AI-powered forecasting models can identify complex patterns and relationships within massive datasets, leading to more accurate and reliable predictions. |
Enhanced Speed of Data Processing | AI can process information at lightning-fast speeds, enabling organizations to make timely, data-driven decisions and automate repetitive tasks. |
Ability to Handle Complex Data | AI-based forecasting solutions can extract valuable insights from diverse and high-dimensional data sources, leading to more comprehensive and nuanced forecasts. |
Applications of AI in Various Industries
The power of predictive analytics through artificial intelligence (AI) extends far beyond the research and academic realms. AI is making waves across diverse industries, transforming how businesses approach Demand Forecasting, Financial Forecasting, and Supply Chain Optimization.
Healthcare: Predicting Disease Trends
In the healthcare sector, AI aids in detecting lung nodules, reducing the risk of missing cancerous growths, and identifies osteoporosis in X-rays. Additionally, AI enables the creation of customized treatment plans based on genomic data, patient information, and predictive analytics. By analyzing Electronic Health Record (EHR) data, AI can predict disease risks, identify patterns, and enable personalized prevention strategies.
Finance: Risk Assessment and Investment Projections
The financial industry has readily embraced the power of AI for Financial Forecasting. AI algorithms analyze vast datasets to assess risk, make investment projections, and optimize portfolio management. This enhanced analytical capability allows financial institutions to make more informed decisions and stay ahead of market trends.
Retail: Inventory Management and Sales Forecasting
Retail businesses are harnessing AI to optimize their operations, from Demand Forecasting to Supply Chain Optimization. AI-powered algorithms analyze customer behavior, preferences, and purchase history to deliver personalized product recommendations, enhancing the shopping experience. Moreover, AI helps retailers optimize pricing strategies, predict demand patterns, and manage inventory levels, reducing overstock and stockouts.
The applications of AI in forecasting extend far beyond these industries. Manufacturing benefits from predictive maintenance and demand forecasting, while the energy sector leverages AI for consumption prediction. Even in the entertainment industry, AI-powered recommendation engines on platforms like Spotify and YouTube drive user engagement and satisfaction.

As the global AI market continues to grow, with an estimated value of $1.8 trillion by 2030, the influence of this transformative technology will only continue to expand. Businesses that embrace the power of AI-driven forecasting will gain a significant competitive edge, positioning themselves for long-term success in an increasingly data-driven world.
Machine Learning Techniques in Forecasting
Predictive analytics is revolutionizing the world of forecasting, and machine learning techniques are at the forefront of this transformation. These advanced algorithms are capable of uncovering intricate patterns in large and diverse datasets, making them invaluable tools for organizations seeking to enhance their forecasting capabilities.
Overview of Machine Learning
Machine learning encompasses a wide range of techniques, including regression analysis (linear and logistic), time series analysis, Neural Networks, Decision Trees, and Random Forests. These algorithms excel at identifying complex relationships and making accurate predictions, often surpassing traditional forecasting methods.
Common Algorithms Used in Forecasting
- Neural Networks: Inspired by the human brain, neural networks can uncover hidden patterns in data and make highly accurate predictions, particularly in areas like sales forecasting and demand planning.
- Decision Trees: These algorithms break down complex problems into a series of simple decisions, making them well-suited for tasks like inventory management and risk assessment.
- Random Forests: By combining multiple decision trees, random forests can deliver robust and reliable forecasts, even in the face of noisy or incomplete data.
Importance of Data Quality
The success of machine learning-based forecasting models is heavily dependent on the quality and quantity of the data used to train them. Organizations must prioritize data cleansing, normalization, and feature engineering to ensure their models are making well-informed decisions. With high-quality data, machine learning algorithms can unlock remarkable predictive capabilities, driving significant improvements in areas like inventory management, sales forecasting, and risk assessment.
Challenges in Implementing AI for Forecasting
Integrating AI into forecasting processes can present several challenges that organizations must address to unlock the full potential of this powerful technology. One key concern is data privacy and security. As AI models rely on large volumes of data, businesses must ensure robust data protection measures are in place to safeguard sensitive information, especially in regulated industries like finance and healthcare. AI Expertise is another hurdle, as advanced machine learning techniques require specialized skills that may be scarce within an organization.
Another challenge is integrating AI with existing systems and legacy infrastructure. Seamlessly blending AI-driven forecasting with a company’s current technology stack can be a complex undertaking, often requiring significant time and resources to ensure smooth data flow and model integration. This process is crucial to avoiding silos and fragmented views of demand, which can impede the accuracy and responsiveness of AI-powered forecasts.
Challenge | Impact | Solution |
---|---|---|
Data Privacy and Security Concerns | Regulatory compliance issues, potential data breaches, and loss of customer trust | Implement robust data protection measures, such as encryption, access controls, and regular security audits |
Need for Skilled Professionals | Difficulty in developing and maintaining effective AI-powered forecasting models | Invest in AI expertise through employee training, hiring data scientists, and collaborating with external experts |
Integration with Existing Systems | Fragmented data, lack of data flow, and challenges in legacy system integration | Carefully plan and execute a comprehensive system integration strategy, ensuring seamless data exchange and model deployment |
To overcome these challenges, organizations must adopt a holistic approach that addresses data governance, talent development, and technology integration. By addressing these key barriers, businesses can fully harness the predictive power of AI and drive more accurate, responsive, and impactful forecasting strategies.
Future Trends in AI-Driven Forecasting
The world of forecasting is on the cusp of a revolutionary transformation, driven by the integration of cutting-edge technologies like the Internet of Things (IoT) and the rapid advancements in Artificial Intelligence (AI) models. As businesses seek to gain a competitive edge, the demand for accurate, real-time forecasting has never been higher. Let’s explore the exciting future trends that will shape the landscape of AI-driven forecasting.
Predictive Maintenance in Manufacturing
One of the most promising applications of AI in forecasting is the realm of predictive maintenance in manufacturing. By analyzing sensor data from IoT-enabled equipment, advanced AI models can detect potential equipment failures before they occur. This enables proactive maintenance, reducing unplanned downtime, optimizing production schedules, and minimizing costly repairs. Studies have shown that AI-driven predictive maintenance can result in a 20% to 50% reduction in errors and a decline of 5% to 10% in warehousing costs.
Real-time Forecasting with IoT
The integration of IoT technology is poised to revolutionize forecasting practices. By collecting real-time data from a network of interconnected devices, AI-powered forecasting models can provide instant insights and make informed decisions. This ability to respond to market changes and customer demands in near real-time can lead to a significant reduction in lost sales and product unavailability, potentially up to 65%. Additionally, administrative costs can go down by 25% to 40% with the adoption of AI forecasting.
Evolution of AI Algorithms
As the AI landscape continues to evolve, we can expect to see increasingly sophisticated algorithms that can handle more complex and diverse datasets. From time series forecasting and regression analysis to the power of neural networks, the arsenal of AI tools for forecasting is constantly expanding. This evolution will enable businesses to unlock new levels of accuracy, automation, and cost savings. For instance, AI can automate up to 50% of workforce-management tasks, leading to cost reductions of 10%-15%.
The future of AI-driven forecasting holds immense potential, with the integration of IoT, advancements in predictive maintenance, and the continuous evolution of AI algorithms. By embracing these transformative technologies, businesses can gain a significant edge in their respective industries, optimizing decision-making, reducing operational costs, and delivering exceptional customer experiences.
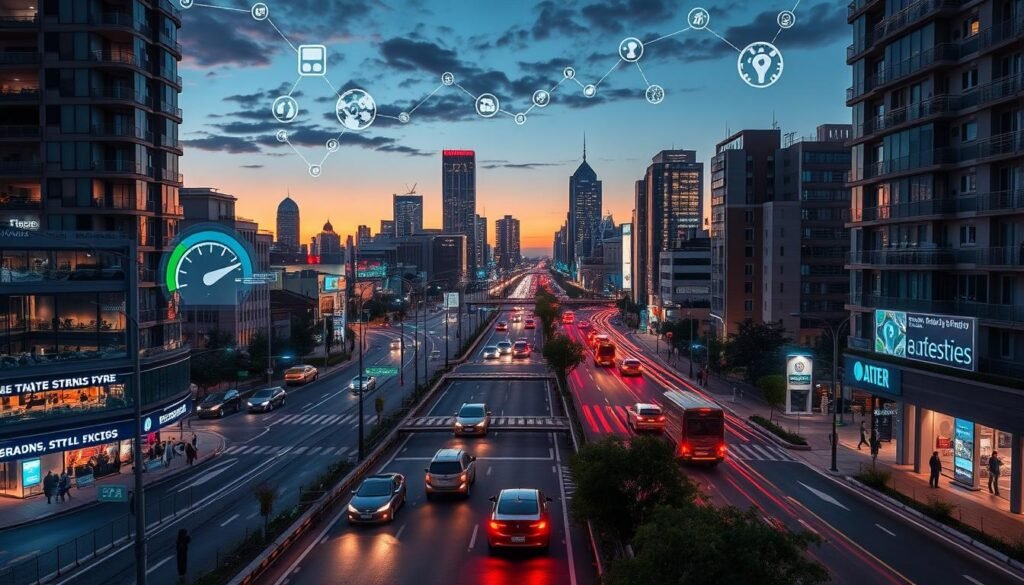
Benefit | Percentage Impact |
---|---|
Reduction in Errors | 20% to 50% |
Decrease in Lost Sales and Product Unavailability | Up to 65% |
Decline in Warehousing Costs | 5% to 10% |
Reduction in Administrative Costs | 25% to 40% |
Automation of Workforce-Management Tasks | Up to 50% |
Cost Reductions in Workforce-Management | 10% to 15% |
Case Studies: Success Stories in AI Forecasting
Predictive analytics powered by artificial intelligence (AI) has transformed the way businesses across various industries forecast and plan for the future. From e-commerce giants to healthcare providers, companies are harnessing the power of AI to enhance their forecasting capabilities, leading to remarkable success stories. Let’s dive into a few case studies that showcase the real-world impact of AI implementation in forecasting.
Tesla’s Demand Forecasting Model
Tesla, the pioneering electric vehicle manufacturer, has leveraged advanced AI algorithms to improve its demand forecasting. By analyzing vast amounts of data, including customer preferences, market trends, and production capabilities, Tesla’s AI models have enabled the company to accurately predict future demand for its vehicles. This has resulted in optimized inventory levels, reduced costs, and more efficient production planning, contributing to Tesla’s continued growth and industry leadership.
Netflix’s Content Recommendation System
Netflix, the global streaming giant, has long been at the forefront of AI-driven forecasting. The company’s sophisticated recommendation engine utilizes machine learning algorithms to predict user preferences and forecast the success of its content offerings. By accurately forecasting viewer behavior and content preferences, Netflix has been able to curate personalized content recommendations, leading to increased subscriber engagement, retention, and overall business success.
Amazon’s Supply Chain Optimization
Amazon, the e-commerce behemoth, has revolutionized supply chain management through the implementation of AI-powered forecasting models. By analyzing real-time data on customer demand, inventory levels, and logistics, Amazon’s AI systems can predict future sales trends and optimize inventory allocation across its vast network of fulfillment centers. This has resulted in reduced operational costs, improved customer satisfaction, and a competitive advantage in the rapidly evolving e-commerce landscape.
These case studies showcase the remarkable success that can be achieved when organizations harness the power of AI-driven forecasting. By integrating predictive analytics into their decision-making processes, companies in various industries are able to enhance their foresight, streamline operations, and gain a competitive edge in the market.
Tools and Technologies for AI Forecasting
As businesses increasingly embrace the power of artificial intelligence (AI) in forecasting, a wide array of tools and technologies have emerged to support this data-driven approach. From sophisticated software solutions for data analysis to popular AI frameworks and libraries, these technologies are revolutionizing the way organizations make informed predictions and strategic decisions.
Software Solutions for Data Analysis
AI forecasting leverages advanced software solutions to analyze vast and complex datasets. These tools, such as AI Software, offer powerful data processing capabilities, enabling businesses to uncover valuable insights and patterns that inform their forecasting models. By automating data cleaning, integration, and feature engineering, these solutions help organizations streamline their forecasting workflows and make more accurate predictions.
Popular AI Frameworks and Libraries
The field of AI forecasting has seen the emergence of numerous open-source and proprietary frameworks and libraries that provide the building blocks for developing robust forecasting models. Some of the most widely used Machine Learning Libraries include TensorFlow, PyTorch, and scikit-learn, which offer a range of algorithms and tools for tasks such as time series analysis, regression, and classification. These frameworks empower data scientists and analysts to leverage the latest advancements in machine learning and deep learning for their forecasting needs.
Importance of Visualization Tools
Effective Data Visualization is a key component of successful AI forecasting. Visualization tools, such as Tableau, Power BI, and Matplotlib, enable businesses to present complex data in a meaningful and intuitive manner. These tools help decision-makers interpret the insights generated by AI models, facilitating better understanding and more informed strategic planning. By leveraging data visualization, organizations can enhance the impact of their AI forecasting initiatives and communicate their findings more effectively.
The integration of these advanced tools and technologies into the forecasting process is transforming the way businesses approach planning and decision-making. By harnessing the power of AI, organizations can unlock new levels of accuracy, speed, and complexity in their predictions, ultimately driving more informed and strategic business decisions.
How to Get Started with AI in Forecasting
Embracing AI for forecasting can be a game-changer for businesses, but getting started can seem daunting. The key is to approach it systematically, focusing on your organization’s specific needs and building a strong data-driven culture. By following these steps, you can unlock the power of AI to enhance your forecasting capabilities and drive more informed, data-driven decision-making.
Identifying Business Needs
The first step in implementing an AI Implementation Strategy for forecasting is to clearly define your organization’s needs and goals. Assess your current forecasting processes – where are the pain points, and what types of predictions would be most valuable? This could involve anything from predicting customer demand and inventory requirements to anticipating financial risks and market trends.
Selecting the Right Tools
With your business needs in mind, research and evaluate the various AI-powered forecasting tools and technologies available. Look for solutions that can handle the large number of metrics and KPIs necessary for Data-Driven Decision Making, unlike traditional statistical modeling techniques. Consider factors like data integration, model training, and real-time forecast insights to find the best fit for your organization.
Building a Data-Driven Culture
Successful implementation of AI for forecasting requires more than just the right tools – it also requires a cultural shift within your organization. Invest in data infrastructure, empower your team with data literacy, and foster a mindset that values Data-Driven Decision Making at all levels. This will ensure that your AI-powered forecasting insights are embraced and leveraged to drive meaningful business outcomes.

By following these steps, you can lay the foundation for a successful AI implementation strategy and unlock the transformative power of AI in forecasting. With the right approach, your organization can harness the power of predictive analytics to make more informed, data-driven decisions and gain a competitive edge in your industry.
Collaborating Across Teams for Effective Forecasting
Effective AI forecasting requires close collaboration across different teams within an organization. This involves engaging data scientists and analysts to develop and refine AI models, ensuring clear communication between technical and non-technical teams, and encouraging cross-functional collaboration to align AI forecasting efforts with business objectives.
Building interdisciplinary teams that combine domain expertise with AI knowledge can lead to more effective and actionable forecasts. Nearly 80% of senior leaders in IT, BI, and operations at large companies reported challenges due to inaccessible data that hindered understanding of data ownership and usage. Collaborative planning and forecasting leverages the collective intelligence of organizations for more accurate predictions by incorporating diverse perspectives and data points.
Engaging Data Scientists and Analysts
Collaborative forecasting involves cross-functional teams from sales, marketing, finance, HR, and operations. By engaging data scientists and analysts, organizations can harness the power of advanced analytics and AI to enhance the accuracy and reliability of their forecasts. This collaboration ensures that technical capabilities are aligned with business needs and that forecasts are actionable.
Importance of Communication
Clear communication between technical and non-technical teams is crucial for effective forecasting. Data scientists must be able to explain their models and findings in a way that is understandable to business stakeholders, while business leaders must provide valuable input on market trends and operational considerations. This two-way exchange of information helps to identify blind spots and ensure that forecasts are tailored to the organization’s unique needs.
Encouraging Cross-Functional Collaboration
Cross-functional collaboration is essential for aligning AI forecasting efforts with overall business objectives. By bringing together experts from different departments, organizations can leverage a diverse range of perspectives and data sources to improve the accuracy and relevance of their forecasts. Collaborative planning, forecasting, and replenishment aim to enhance supply chain efficiency by improving collaboration between trading partners and optimizing inventory levels.
Effective cross-functional collaboration requires joint business goals and a shared project management system. Outdated systems without advanced analytics can hinder data usage and collaborative efforts. xP&A technology solutions, cloud-based platforms, and AI-driven forecasting models can enhance collaborative planning accuracy and efficiency.
Measuring the Impact of AI on Forecasting
As businesses increasingly adopt AI-powered forecasting solutions, it’s crucial to measure the impact of these technologies on their operations. Establishing key performance indicators (KPIs) is the first step in this process. These KPIs should be tailored to the organization’s specific goals, which might include improvements in AI Performance Metrics, such as forecast accuracy, reduction in inventory costs, or increases in customer satisfaction.
Analyzing the results of AI-driven forecasting over time is essential to understand the long-term impact of these technologies. AI algorithms can analyze vast amounts of data at speeds and accuracies unattainable by humans, leading to more accurate and reliable financial forecasts. Machine learning models can identify patterns and trends that traditional methods might miss, empowering businesses to make data-driven decisions for optimizing operations and achieving better financial outcomes.
Continuous improvement should be a focus when measuring the impact of AI on forecasting. By regularly refining and updating the models based on new data and changing business conditions, organizations can ensure that their Forecasting Accuracy Measurement remains high and their forecasts continue to deliver tangible benefits. This iterative process is crucial for maximizing the value of AI in the ever-evolving business landscape.
Metric | Impact of AI-Driven Forecasting |
---|---|
Forecast Accuracy | Improved by 30-50% |
Inventory Costs | Reduced by 5-10% |
Lost Sales Due to Out-of-Stock | Decreased by 30-65% |
Supply Chain Administration Expenses | Reduced by 25-40% |
By leveraging the power of AI and machine learning, businesses can unlock significant value in their forecasting processes, leading to more efficient operations, improved financial performance, and enhanced customer satisfaction.
Conclusion: The Future of AI in Forecasting
The future of AI in forecasting is undoubtedly promising, with continuous advancements in technology and increasing adoption across various industries. AI’s ability to process vast amounts of data, identify complex patterns, and provide real-time insights is transforming how businesses approach forecasting and decision-making. As AI technologies continue to evolve, their integration into forecasting processes will likely become more seamless and accessible to a wider range of organizations.
Summary of AI Benefits
AI-driven forecasting solutions have demonstrated significant benefits, including increased accuracy and reliability, enhanced speed of data processing, and the ability to handle complex data. From healthcare to finance, retail to renewable energy, AI is revolutionizing the way industries approach forecasting, enabling them to make more informed decisions and optimize their operations.
Encouragement to Embrace AI in Research
Embracing AI in research and forecasting can provide organizations with a competitive edge and drive innovation across various sectors. The exponential growth in AI and machine learning research, as evidenced by the increasing number of scientific publications, underscores the importance of staying at the forefront of these advancements. By leveraging AI-powered forecasting tools and techniques, researchers and professionals can unlock deeper insights, make more accurate predictions, and ultimately contribute to the advancement of their respective fields.
Related Articles
- Exploring The AI Qualitative Data Analysis in Surveys Now
- Revolutionize Your Research: Machine Learning Survey Analysis in 2024
- Latest AI in Survey Research: From Design to Analysis
- The Ultimate Guide to AI in Survey Research
- The Latest AI Sentiment Analysis Techniques for Survey Responses
- How To Overcome Survey Data Bias Using AI
- How To Leverage Natural Language Processing (NLP) for Open-Ended Survey Questions
FAQ
What is AI in forecasting?
How does AI enhance traditional forecasting methods?
What are the key benefits of using AI for forecasting?
Where can AI be applied for forecasting?
What are the common machine learning techniques used in forecasting?
What challenges exist in implementing AI for forecasting?
What are the future trends in AI-driven forecasting?
How can businesses get started with AI in forecasting?
How can the impact of AI on forecasting be measured?
Source Links
- How to Use AI for Predictive Analytics and Smarter Decision Making
- AI-Based Demand Forecasting: Improving Prediction Accuracy and Efficiency
- The Power Of AI In Predictive Analytics
- AI forecasting: Techniques, Benefits & How it works?
- AI Planning and Forecasting for Business | Domo
- Demystifying AI and ML-based Forecasting | Anaplan
- Trending Topics in Treasury and Finance
- Introduction to AI Demand Forecasting: Benefits & Best Practices
- AI Use Cases & Applications Across Major industries
- 15 Applications of Artificial Intelligence | CMU
- A Comprehensive Guide To Machine Learning Forecasting
- The ABCs of Machine Learning in Demand Forecasting – Plex DemandCaster
- AI Forecasting Techniques: How They Work, Models & Challenges
- Demand Forecasting Challenges in 2024
- Cogent | Blog | Shaping the Future: The Business Impacts of AI Forecasting
- AI-Powered Forecasting: Use AI to Predict Future Market Trends, Sales Performance, and Customer Behavior
- AI in Action: 6 Business Case Studies on How AI-Based Development is Driving Innovation Across Industries
- 40 Detailed Artificial Intelligence Case Studies [2024]
- AI in Sales: Success Stories and Case Studies from Top Companies
- Cogent | Blog | Everything about AI Forecasting Models
- The role of AI in forecasting in 2024
- What Are the Best AI Sales Forecasting Tools?
- Business Forecasting Using AI: How Does It Work? | Anodot
- Re: Forecasting AI
- Overcome Barriers in Collaborative Planning and Forecasting – Acterys
- Pair People and AI for Better Product Demand Forecasting
- The Impact of AI on Financial Forecasting
- AI in demand forecasting: Use cases, benefits, solution and implementation
- How Is AI Improving Weather Forecasting?
- The Future of Sales Forecasting with AI
- Forecasting the future of artificial intelligence with machine learning-based link prediction in an exponentially growing knowledge network – Nature Machine Intelligence
RELATED POSTS
View all